On October 22nd, 2020, as part of ICTC’s Technology and Human Right Series, Rosina Hamoni, Research Analyst with ICTC, interviewed Dr. Melissa McCradden, Bioethicist with The Hospital for Sick Children and Assistant Professor at the University of Toronto. Rosina interviewed Dr. McCradden about her role as a bioethicist, explainable AI, algorithmic fairness in building ML models, and the future of our healthcare system.
Technology plays an increasingly important role in all sectors, and particularly in the healthcare sector. COVID-19 has amplified the need to accelerate healthcare technology. So, a discussion on the ethics of technology is a natural complement to these developments.
Rosina: Thank you for joining me today, Dr. McCradden! It’s a pleasure to speak with you. I know you have a varied background — spanning psychology, neuroscience, and bioethics — but I’m wondering what sparked your interest in bioethics?
Dr. McCradden: It’s definitely not a linear path. I’ve always been interested in people; I started with psychology and ended up going into neuroscience. My PhD was in youth and concussions. Neuroscience is hard, but what I found much harder actually were the ethical questions that I encountered during this time. For example, we can ask questions like “how do we balance a child’s need to let their brain heal while also mitigating psychosocial risks from them sitting out from a concussion, missing out on activities” and “should we allow them to take part in concussion research?” and “how should we weigh the word of an athlete who is so desperate to get back to their sport when they swear they have no concussion symptoms. What I really love about bioethics is that it’s a field where you get to integrate knowledge about people, science, and philosophy to try to make the best possible decisions in really challenging situations. Paediatric bioethics, in particular, is where I always wanted to be because I really value the opportunity to think through how we promote the flourishing of children and young people. I’m really glad that at SickKids, everything that we do is geared toward making the world better for children.
Rosina: Could you expand a bit on your research and interests as a bioethicist at SickKids?
Dr. McCradden: As a Bioethicist at SickKids, I conduct clinical and organizational consultation, research, education, and policy for both AI and non-AI issues. A lot of what I do is clinical. I connect with clinical teams, attend rounds, and provide support for staff who are faced with ethically challenging situations. On the research side, I focus on ethical issues with respect to AI and precision medicine. One area in particular is explainability. We’re working with colleagues at the Vector Institute in addition to staff at SickKids in the cardiac intensive care unit (ICU) and emergency department on an explainability project involving computer scientists, clinicians, and myself — bringing in a bioethicist perspective. Explainability is very complex and the concept has evolved quite significantly in a short time. Our research investigates how we should proceed with explainability. What I mean by this is, recognizing the accountability that is needed, but without resulting in another type of bias with respect to clinical decision making. So, it’s really important that we explore what the actual clinical need is, what is computationally feasible, and what is ultimately going to facilitate the best use of AI to improve the care of patients.
Rosina: Your article, “What Clinicians Want: Contextualizing Explainable Machine Learning for Clinical End Use” focuses specifically on explainability, or “explainable AI.” Could you speak a bit about this emerging field?
Dr. McCradden: Explainability’s landscape has shifted. Initially, it was thought of as a means to address the idea that clinicians need and want to understand the outputs or the predictions of an AI system. Explainability itself is the computational process of providing an account of why or how a prediction was made in a particular case, or it can also provide an overall account of how the model operates. It’s become a bit questionable because emerging evidence is indicating that we might put a little bit too much stock in those explanations themselves. And I think that part of the reason for this is that we as humans tend to think of explanations in a certain way, and that’s not entirely in sync with what computational “explanation” is achieving. It seems to be that when an AI system makes a prediction about a particular outcome that we may be anticipating, and that prediction is accompanied by an explanation, humans tend to make a logical jump into thinking that the system is explaining what’s actually happening in the real world. But the “explanation” is about the model, not about the real world, though these can align. For example, if you have a watch that purportedly detects your stress levels, you’ve probably noticed that sometimes it’s not detecting stress, it’s detecting something else, such as an elevated heart rate. Although it could probably show you the data that it was drawing from to make the prediction, it’s not always “right” in the sense that it’s correctly detecting your stress versus something else.
Rosina: What ethical advantages and drawbacks arise out of explainable AI in healthcare?
Dr. McCradden: Originally, I think many thought that the errors AI would make would be very obvious and easily dismissed by clinicians with the help of explainability. Recent research testing the use of explainability solutions has shown that sometimes an explanation might persuade a clinician to change their mind even when that explanation is wrong. This is particularly evident when there is medical uncertainty. In many ways this makes sense. If you’re a clinician and you’re interpreting an image, for example, and it’s really obvious that the AI system is wrong about there being a presence of an abnormality, you’re going to quickly reject that prediction. But if you’re not sure yourself and you see an explanation that seems convincing, it may seem reasonable to go with the computer output because it might be picking up on something that you are missing. Sometimes it is reasonable, and it is through research that we can demonstrate whether, how, and in what context the AI system is better than the human eye. But over-trust can also lead to unintended consequences, so we’re doing research to try and figure out how we should go about balancing the need for understanding of the AI tool with the desire to avoid this new form of bias.
We want explanations for the purposes of informed consent as well. Ethically, clinicians must provide their reasoning for medical recommendations to support patient and substitute decision-making. But clinicians don’t need all the information about a model to do this. For example, they don’t need to know all the technology behind how an MRI generates images to explain the benefits and risks of MRIs to a patient. The same is true for AI, but we haven’t quite sorted out exactly what information does need to be provided in a given case. I think this is a key place for engagement between computer scientists, patients, parents, clinicians, and regulators to sort this out and I hope our research will support that discussion.
Rosina: In another article, “Ethical limitation of algorithmic fairness solutions in healthcare machine learning,” you mention that algorithmic fairness has not previously incorporated causal relationships. Social determinants of health really surfaced in the early 2000s. As you mention in the article, factors of social determinants of health are key to account for in models.
For our readers who may be unfamiliar with the idea of social determinants of health, could you expand a little on what they are and what this means for machine learning in health care?
Dr. McCradden: Social determinants of health are the economic and social factors that can impact someone’s health status. However, they may not necessarily cause particular health problems in and of themselves. For example, structural racism has greatly impacted the lives of Black and Indigenous people and people of colour, often shaping who lives where, under what conditions, what opportunities people have in society, among other factors. Scholars like Dorothy E. Roberts and Kimberlé Williams Crenshaw have revealed how these factors have implications for health across a number of different pathways, which all intersect.
From an AI lens, we see these effects come up in the apparent differences in the system’s performance as a function of peoples’ identities (e.g., racial identity, gender). The AI is effectively [reflecting] patterns of unfairness that exist in the world, and [it] sometimes has incomplete knowledge or understanding of how these patterns came about. The challenge for health AI is that the clinician and patient will want an accurate assessment of the patient’s clinical status or prognosis — but ideally uninfluenced by the effect of social factors that may not be related to the health problem itself. For many health problems though, we don’t have perfect knowledge of their relationships to social determinants of health.
Dorothy E. Roberts says that many times we create shortcuts where the observation that a condition is more common among one racial group turns into a mistaken belief that a risk for that condition is inherent to that population and that identity. AI developers don’t have the knowledge of the complexity of these relationships. But clinicians don’t have all the knowledge about them either. In our paper, we stress the importance of looking to anthropologists, critical race scholars, intersectional researchers, and others to get a better understanding of what’s going on.
Rosina: To ensure that the social determinants of health are accounted for fairly in healthcare algorithms, what advice would you provide to ensure these sorts of biases are accounted for?
Dr. McCradden: I think the most ethical approach depends on a number of factors, in addition to what the algorithm itself is intended to do. It also depends on what we mean by “accounting for” these biases. Sometimes AI can help reveal biases so that we can take action to fix the real problem. In other cases, we should know someone’s social determinants of health so we can better direct resources to them or find solutions that are aimed at these determinants. If we corrected for these factors, we might miss this important insight. We always need to take steps to make sure that actions do not disadvantage a person experiencing some structural vulnerability, be it housing, poverty, or others.
Clearly, the most ethically justifiable way of accounting for bias is to first determine the impact you’re intending the algorithm to have. For example, if referral rates between patients of different identities are not equivalent and there’s no medical reason for this, an algorithm can help ensure that the referral rates are fairer. In other cases, you can change the algorithm to work on a slightly different aspect of the problem.
What we really stressed in the paper was that you need to have strong knowledge about the problem being modelled, the task that you’re intending to address, and the prioritization of the intended ethical goal. These biases are in us and in the world, and so they’re in the algorithm too. The most important thing is to prioritize the structural solutions put forward by many scholars because this will lead to equity in the world, which would then be reflected in algorithms.
Rosina: AI, being one of the most promising technologies, can certainly help solve some societal challenges, although in so doing can also pose ethical challenges. I’m wondering what you would consider to be a main challenge that we are currently facing in regard to AI and health, beyond the examples you have already given?
Dr. McCradden: I think there’s a lot of perennial ethics issues that take on a different kind of angle with AI. It is new, but it’s not always entirely unique. And I think one of the main challenges that we have is that we in healthcare operate in a space where we have particular obligations to patients. So, we don’t have the “move fast and break things” model because it’s not things you would be breaking, it’s people. We’re slow and deliberate, ideally. But we also don’t want to be so slow that we’re not moving things forward because ultimately there can be immense benefit from the use of some of these algorithms.
One of the big things that I’d love to change is this idea that an ethical framework is the endpoint of thinking about ethical issues. If you think about other technological advances in medicine, like ventilators or surgery, we have ongoing discussions about the ethics of these interventions, under what conditions, and why. And I think it’s no different for AI, the ethics of it lives in the daily interpersonal and socio-technical interactions that we have in each instance of use of the technology. So, I think that the ethics of AI, rather than being one end point in the development pipeline, will actually be a continuous, ongoing. and evolving discussion.
Rosina: How do you think technology, beyond AI, is impacting healthcare system?
Dr. McCradden: I think for all of human history, technology has always kind of prompted a range of reactions that run the full spectrum. And I really like this work by Madeleine Claire Elish, who was part of the Duke team who developed Sepsis Watch. She talks about something called repair work, the idea that there’s adjustments that we make to compensate for a change. Every time we have a new technology, it disrupts our current workflows, and it takes time before they’re seamlessly integrated. Elish doesn’t mean “disrupts” in a negative way, she just points out that change is accompanied by certain repair work where we have to adjust and adapt. For example, if you look at the adoption of video conferencing technologies during the COVID-19 pandemic, it’s enabled us to get together and accomplish things while keeping ourselves safe. But we also have the big problem of video conferencing fatigue. And so now, again, we start making adjustments to how we can make this work better. So, digital health, I think, has a lot of great advantages, but the trick is to get that calibration right so that you end up with a net overall good effect.
Rosina: To conclude, I’m wondering what you feel most excited about in relation to a digital-first future, and how it will impact our healthcare system?
Dr. McCradden: I think that AI can enable us to do things much more consistently and with better accountability and documentation. I think it is all in this period of flux, but the greatest advantage of computers is that they are consistent. Humans do make mistakes and have biases, and computers do too, but they’re different kinds of biases and mistakes. So, if you put them together, you are aiming to have this net overall good effect. But I think that’s why ethics is essential; because we don’t know everything and yet we want to make the best decisions we can in a defensible manner. I think one of the best things, in my view, is that AI has tested just about every boundary of these dimensions of decision-making, from clinical care to knowledge about knowledge, so its introduction into healthcare is making us think harder and more critically and overall better than before. I think beyond the advantages of getting more information, reducing errors, and enabling anticipation, AI is enhancing the quality of our thinking within healthcare and practice overall.
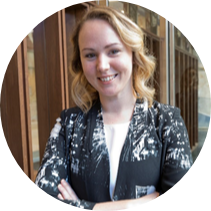
Dr. McCradden is a Bioethicist and a Project Investigator with the Genetics & Genome Biology program at The Hospital for Sick Children (SickKids). She is an Assistant Professor with the Division of Clinical and Public Health at Dalla Lana School of Public Health at the University of Toronto. She holds a PhD in Neuroscience, a Master’s in Bioethics, and she was the inaugural postdoctoral fellow in the Ethics of AI in Healthcare with SickKids and Vector Institute. Her research focuses on paediatric bioethics and the ethics of healthcare machine learning, AI, and precision medicine.